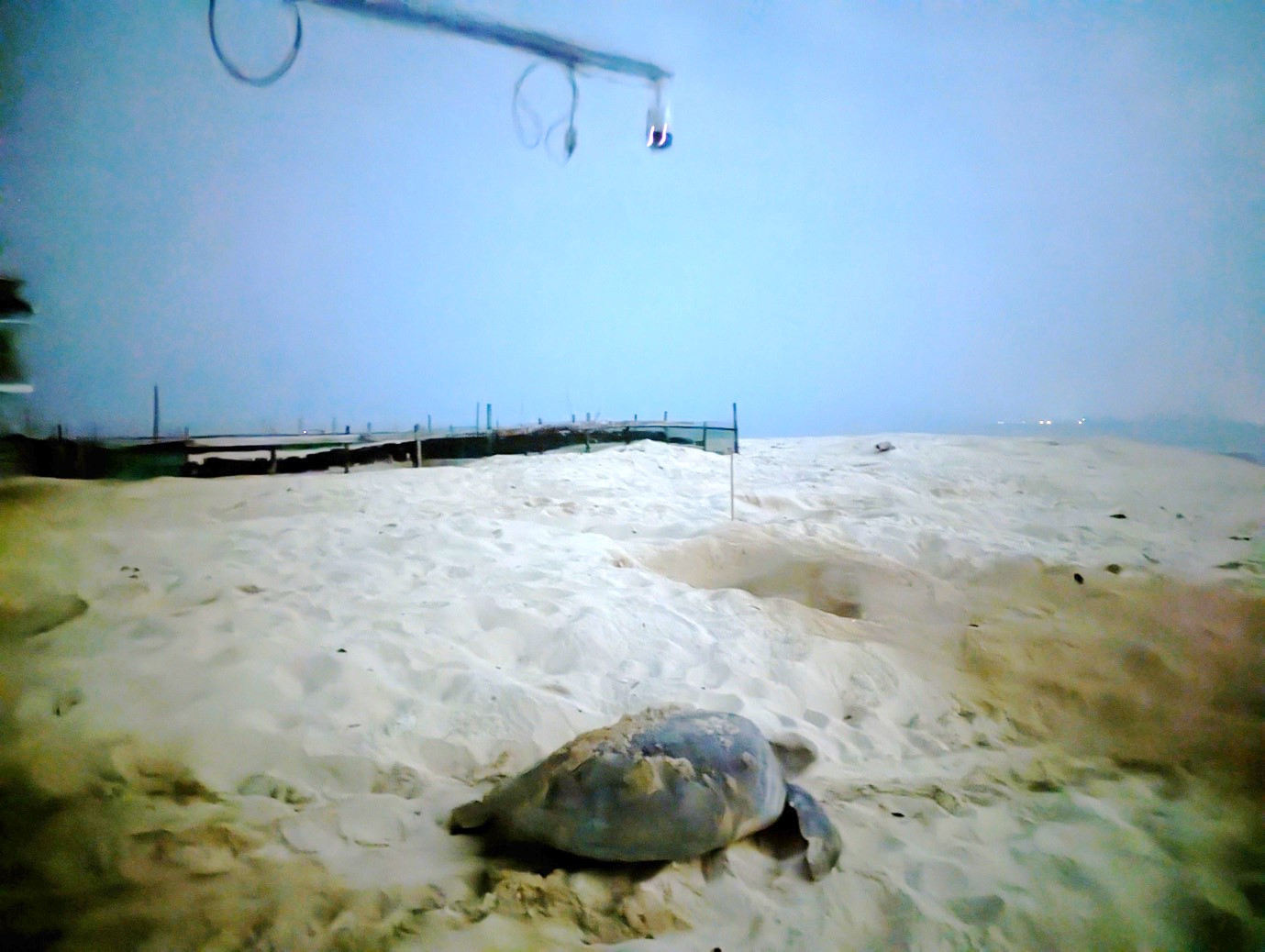
Located on the Island of Borneo, Sarawak is blessed as a home to a diverse range of marine life, including the iconic Green sea turtle (Chelonia mydas).
These magnificent creatures are a vital part of Sarawak’s coastal ecosystem and are known for their significant role in maintaining the health of coral reefs and seagrass beds of the state’s surrounding waters.
In Sarawak, these migratory animals are primarily found nesting on remote sandy beaches, where they lay their eggs in large nests dug in the sand.
Known as natal homing, the female sea turtle makes the long trip back to her natal beach to lay her eggs.
Under the custodianship of Sarawak Forestry Corporation (SFC), several such sites are declared as Totally Protected Areas (TPA) to reduce human contact with these animals, thus boosting their population size.
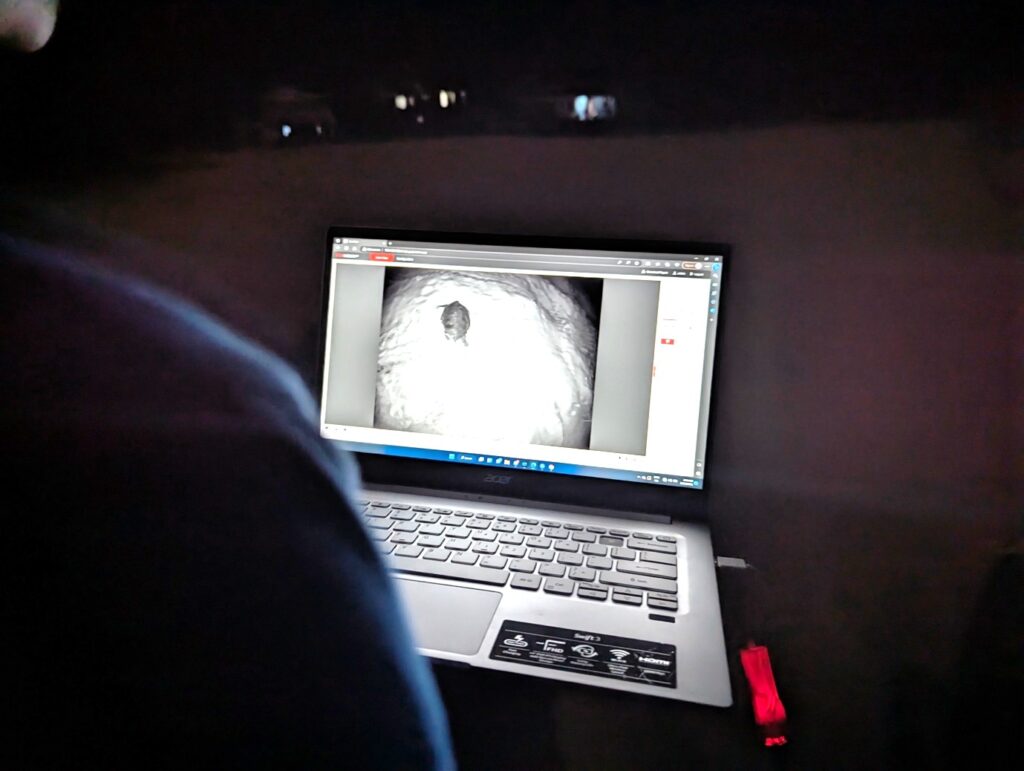
A research team from UNIMAS and SFC has collaborated on a project to develop a tagless/markerless re-identification prototype for female Green sea turtles.
Instead of using plastic/metal tags, the team explored the use of a downward-facing camera that captures images of the nesting individuals.
Similar to human fingerprints, individual animals can be identified via biometric markings.
For green sea turtles, the unique scute patterns on their carapace can be used to distinguish individuals. This human-less approach reduces stress on the Green sea turtles since there is no need for a mark-recapture process nor artificial tagging.
Since Chelonia mydas is a long-lived and migratory animal, the carapace undergoes physical changes due to ageing and external factors.
Khalif Amir Zakry and Syahiran Soria, two MSc students (by research) from the Faculty of Computer Science and Information Technology (FCSIT), were tasked to design and develop Artificial Intelligence (AI) methods that specifically address these issues.
Syahiran trained a custom deep learning model that performs automated Region-of-Interest (ROI) cropping of the carapace from still images.
Also using Deep learning, Khalif integrated the trained model with attention mechanism to direct the deep network “where to look” for the most stable salient features over time.
The models were trained on an image dataset collected at Talang-Satang island over the period of two years.
Both students have had their research articles accepted in SCOPUS-indexed journals awaiting publication.
Although there is still much work to be done to improve the working prototype, the team is pleased to have made a contribution toward the preservation of these magnificent creatures and their fragile ecosystems.
Future work will involve extending the duration of the data collection period and rigorously validating the proposed methods against a much larger dataset.
If you are interested to know more about this research work, please contact Dr Irwandi Hipiny at the following email address: mhihipni@unimas.my.
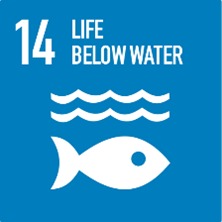
This article is related with Sustainable Development Goal (SDG 14) – Life Below Water